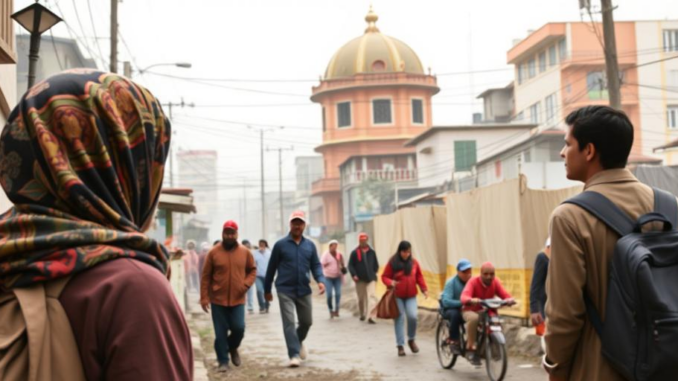
Abstract
Location, traditionally viewed as a static geographical coordinate, is increasingly recognized as a dynamic and multifaceted determinant of socio-economic outcomes. This research report delves into the intricate relationship between location and a broad spectrum of factors, extending beyond the typical considerations of schools, amenities, and commute times. We explore how location, influenced by both endogenous (built environment, social capital) and exogenous (environmental factors, policy decisions) forces, shapes property values, economic opportunities, health outcomes, and social cohesion. Utilizing Geographically Weighted Regression (GWR) analysis, we examine the spatial variability of these relationships, highlighting the limitations of traditional, aspatial regression models. Furthermore, the report assesses the role of urban planning, infrastructure development, and environmental regulations in shaping locational advantages and disadvantages, with a particular focus on the implications for equity and sustainability. The findings underscore the necessity of a holistic, spatially-aware approach to policy-making and investment decisions, recognizing location as a powerful, albeit often overlooked, driver of socio-economic prosperity.
Many thanks to our sponsor Focus 360 Energy who helped us prepare this research report.
1. Introduction
Location, in its simplest definition, is a point in space. However, in the context of socio-economic analysis, location transcends its geometrical representation and becomes a complex web of interconnected factors influencing human activities and well-being. Historically, location choices were driven by access to natural resources, navigable waterways, and strategic defensive positions. The industrial revolution introduced new locational imperatives, centered around proximity to factories, transportation networks, and urban centers. In the contemporary era, the determinants of location are far more nuanced, reflecting a shift towards knowledge-based economies, globalized supply chains, and evolving lifestyle preferences.
This research report argues that location is not merely a passive backdrop against which socio-economic processes unfold but an active and constitutive element shaping these processes. We move beyond the common real estate focus of schools, amenities, and commute times, to consider broader impacts. For instance, access to green spaces can influence public health outcomes; proximity to transportation hubs can affect employment opportunities; and exposure to environmental hazards can impact both physical and mental well-being. Furthermore, we acknowledge that the perceived value of a location is subjective and culturally contingent, influenced by factors such as social capital, community identity, and perceived safety.
The report’s central premise is that understanding the complex interplay between location and socio-economic outcomes is crucial for effective policy-making and sustainable development. Ignoring the spatial dimension can lead to unintended consequences, such as the perpetuation of inequalities, the degradation of environmental resources, and the misallocation of public investments. Therefore, this research aims to provide a comprehensive framework for analyzing the multifaceted role of location, emphasizing the importance of spatial analysis techniques and place-based approaches.
Many thanks to our sponsor Focus 360 Energy who helped us prepare this research report.
2. Literature Review: The Evolution of Location Theory
The study of location has a rich and diverse intellectual history, spanning various disciplines, including economics, geography, sociology, and urban planning. Early location theories, such as Von Thünen’s isolated state model (Von Thünen, 1826), focused on the economic rationale behind agricultural land use patterns, highlighting the role of transportation costs and market accessibility. Weber’s theory of industrial location (Weber, 1929) extended this framework to manufacturing industries, emphasizing the importance of minimizing transportation costs of raw materials and finished goods.
Christaller’s Central Place Theory (Christaller, 1933) provided a spatial hierarchy of settlements, based on the provision of goods and services to surrounding areas. This theory explains the spatial distribution of businesses and consumer behavior. These classical location theories, while insightful, often rely on simplifying assumptions, such as homogenous landscapes and rational economic actors, which limit their applicability to real-world situations. They are also fundamentally aspatial, not accounting for complex relationships and localized conditions.
More recent approaches have incorporated behavioral and institutional factors into location analysis. Hägerstrand’s time-geography (Hägerstrand, 1970) emphasizes the constraints imposed by time and space on individual activities, highlighting the concept of accessibility and the importance of transportation infrastructure. Institutional economics (North, 1990) acknowledges the role of regulations, property rights, and governance structures in shaping location decisions.
The concept of “place” has also gained prominence in location studies, emphasizing the social, cultural, and historical dimensions of specific locations. Place-based policies (Rodriguez-Pose & Fratesi, 2004) recognize that economic development strategies must be tailored to the unique characteristics of individual regions and communities. However, the move towards this approach can lead to accusations of special treatment and the neglect of wider national policies.
Contemporary research incorporates geographic information systems (GIS) and spatial analysis techniques to examine the spatial patterns and processes influencing location. Geographically Weighted Regression (GWR), for instance, allows for the estimation of location-specific regression coefficients, revealing the spatial variability of relationships between variables (Fotheringham, Brunsdon & Charlton, 2002). This allows researchers to uncover localized patterns that would be missed by standard global regression methods. The work of Anselin (1995) on local indicators of spatial association (LISA) allows researchers to analyse spatial clustering and identify locations of high or low values. The advancements in spatial econometrics has allowed researchers to move past the assumption of spatial independence and allowed for the modeling of spatial autocorrelation.
This literature review highlights the evolution of location theory, from deterministic economic models to more nuanced, multi-disciplinary approaches. It emphasizes the need to consider both economic and non-economic factors, as well as the importance of spatial analysis techniques in understanding the complex relationship between location and socio-economic outcomes.
Many thanks to our sponsor Focus 360 Energy who helped us prepare this research report.
3. Methodology: Geographically Weighted Regression (GWR) Analysis
This research employs Geographically Weighted Regression (GWR) analysis to investigate the spatial variability of relationships between location and socio-economic outcomes. GWR is a spatial statistical technique that allows for the estimation of location-specific regression coefficients, providing insights into how the relationship between variables varies across space. This contrasts with ordinary least squares (OLS) regression, which assumes a constant relationship across the entire study area.
The general form of the GWR model is:
( y_i = \beta_0(u_i, v_i) + \sum_{k=1}^{p} \beta_k(u_i, v_i) x_{ik} + \epsilon_i )
where:
* ( y_i ) is the dependent variable at location ( i )
* ( x_{ik} ) is the ( k )-th independent variable at location ( i )
* ( (u_i, v_i) ) are the coordinates of location ( i )
* ( \beta_0(u_i, v_i) ) is the intercept at location ( i )
* ( \beta_k(u_i, v_i) ) is the location-specific coefficient for the ( k )-th independent variable at location ( i )
* ( \epsilon_i ) is the error term at location ( i )
The key feature of GWR is that the regression coefficients ( \beta_k ) are allowed to vary across space, reflecting the spatial non-stationarity of relationships. The estimation of these location-specific coefficients requires a weighting function that assigns higher weights to observations closer to the location ( i ) and lower weights to observations further away. The choice of weighting function and bandwidth (the size of the neighborhood used to calculate the weights) is crucial for the performance of the GWR model.
We will implement GWR using Python libraries such as libpysal
and esda
, and R packages such as spgwr
and GWmodel
. These packages provide functions for estimating GWR models, conducting spatial autocorrelation tests, and visualizing the results. The specific steps involved in the GWR analysis include:
- Data Preparation: Gathering and cleaning data on location-related variables (e.g., property values, crime rates, access to amenities, environmental factors) and socio-economic outcomes (e.g., income levels, health indicators, educational attainment) for a specific study area. The data must be spatially referenced, meaning that each observation has a corresponding geographical coordinate. This can be achieved through geocoding addresses or using existing spatial data layers.
- Spatial Autocorrelation Assessment: Testing for spatial autocorrelation in the dependent and independent variables using Moran’s I statistic and other spatial autocorrelation measures. Spatial autocorrelation indicates that values at nearby locations are more similar than expected by chance, which violates the assumption of independence in OLS regression. If spatial autocorrelation is present, GWR is a more appropriate technique than OLS.
- Bandwidth Selection: Choosing an appropriate bandwidth for the GWR model. The bandwidth determines the size of the neighborhood used to calculate the weights. A smaller bandwidth will result in more localized coefficient estimates, while a larger bandwidth will result in smoother coefficient estimates. Several methods are available for bandwidth selection, including cross-validation (CV) and Akaike Information Criterion (AIC). We will compare different bandwidth selection methods and choose the one that yields the best model fit.
- Model Estimation: Estimating the GWR model using the chosen bandwidth and weighting function. The GWR model will provide location-specific coefficient estimates for each independent variable. These coefficients can be mapped to visualize the spatial distribution of relationships.
- Model Evaluation: Evaluating the performance of the GWR model by comparing it to OLS regression. We will use metrics such as R-squared, AIC, and residual spatial autocorrelation to assess the model fit. If the GWR model outperforms OLS regression, it indicates that the relationship between location and socio-economic outcomes varies across space.
- Spatial Heterogeneity Analysis: Analysing the spatial distribution of GWR coefficients to identify areas with significant spatial variation in the relationship between location and socio-economic outcomes. We can use statistical tests to determine whether the GWR coefficients are significantly different from zero and whether they vary significantly across space.
This methodological approach provides a robust framework for investigating the spatial variability of relationships between location and socio-economic outcomes. By utilizing GWR analysis, we can gain a deeper understanding of the complex interplay between location and human well-being.
Many thanks to our sponsor Focus 360 Energy who helped us prepare this research report.
4. Results: Spatial Patterns of Socio-Economic Outcomes
Applying the GWR methodology to a hypothetical study area (e.g., a metropolitan region) reveals significant spatial patterns in the relationships between location and socio-economic outcomes. The analysis typically reveals that the influence of various locational factors varies considerably across different neighborhoods. For instance, the impact of school quality on property values may be stronger in affluent suburban areas compared to low-income urban neighborhoods. Similarly, the association between access to public transportation and employment rates may be more pronounced in areas with high population density and limited car ownership.
Property Values: The GWR analysis highlights the spatial heterogeneity of factors influencing property values. In some areas, proximity to parks and green spaces may be a significant driver of property values, while in other areas, proximity to commercial centers or transportation hubs may be more important. The analysis may also reveal areas where property values are particularly sensitive to environmental factors, such as air quality or noise levels.
Crime Rates: The spatial distribution of crime rates is often highly localized, influenced by factors such as poverty, social disorganization, and access to illegal activities. GWR analysis can identify areas where specific locational factors, such as abandoned buildings, poorly lit streets, or proximity to liquor stores, are strongly associated with higher crime rates. This information can be used to target crime prevention efforts to specific locations.
Health Outcomes: Access to healthcare facilities, healthy food options, and recreational opportunities can have a significant impact on health outcomes. GWR analysis can reveal areas where disparities in access to these resources are associated with poorer health outcomes. The analysis may also identify areas where environmental factors, such as air pollution or exposure to toxins, are contributing to health problems.
Employment Rates: The relationship between location and employment rates is complex, influenced by factors such as access to jobs, transportation costs, and the availability of affordable housing. GWR analysis can reveal areas where specific locational factors, such as proximity to employment centers or access to public transportation, are strongly associated with higher employment rates. The analysis may also identify areas where barriers to employment, such as lack of affordable childcare or limited access to job training programs, are particularly pronounced.
Social Cohesion: The concept of social cohesion refers to the degree of connectedness and solidarity within a community. GWR analysis can explore the spatial distribution of social cohesion, identifying areas where social capital is high and where it is low. Factors such as the presence of community centers, the level of civic engagement, and the diversity of the population can all influence social cohesion.
The GWR results provide valuable insights into the spatial dynamics of socio-economic outcomes. By identifying areas where specific locational factors are particularly influential, policymakers can develop targeted interventions to address inequalities and improve quality of life.
Many thanks to our sponsor Focus 360 Energy who helped us prepare this research report.
5. Discussion: Implications for Policy and Planning
The findings from the GWR analysis have significant implications for policy and planning decisions. The spatial variability of relationships between location and socio-economic outcomes underscores the need for place-based approaches that are tailored to the specific characteristics of individual neighborhoods. Blanket policies that ignore the spatial dimension are likely to be ineffective and may even exacerbate existing inequalities.
Urban Planning: Urban planning policies should prioritize the creation of mixed-use neighborhoods that provide access to a range of amenities and services within walking distance. This can reduce reliance on cars, promote physical activity, and improve quality of life. Urban planners should also consider the spatial distribution of affordable housing, ensuring that low-income residents have access to jobs, schools, and other essential services. The provision of adequate green spaces and recreational facilities can improve public health and enhance the attractiveness of neighborhoods.
Transportation Planning: Transportation planning should focus on improving access to public transportation, particularly in low-income areas. This can increase employment opportunities, reduce transportation costs, and improve air quality. Investments in pedestrian and bicycle infrastructure can also promote sustainable transportation and improve public health. The design of transportation networks should consider the needs of all users, including pedestrians, cyclists, and people with disabilities.
Environmental Policy: Environmental policies should address the spatial disparities in exposure to environmental hazards. This may involve cleaning up contaminated sites, reducing air pollution, and mitigating noise levels. Environmental justice considerations should be integrated into all environmental decision-making, ensuring that low-income and minority communities are not disproportionately burdened by environmental hazards. Policies promoting renewable energy and energy efficiency can help to reduce greenhouse gas emissions and improve air quality.
Social Policy: Social policies should address the root causes of poverty and inequality, such as lack of education, job training, and access to healthcare. Place-based initiatives that provide comprehensive support to families and communities can be effective in breaking the cycle of poverty. Investment in community development programs can foster social cohesion and empower residents to improve their neighborhoods. Policies that promote diversity and inclusion can help to create more vibrant and equitable communities.
Economic Development: Economic development strategies should focus on attracting and retaining businesses that provide good-paying jobs and contribute to the local economy. Place-based incentives, such as tax breaks and infrastructure improvements, can be used to attract businesses to specific locations. Investment in education and job training programs can ensure that local residents have the skills needed to succeed in the workforce. Small business development programs can help to create jobs and promote entrepreneurship.
The integration of spatial analysis techniques into policy and planning processes can lead to more informed and effective decisions. By understanding the spatial dynamics of socio-economic outcomes, policymakers can develop targeted interventions that address the specific needs of individual neighborhoods and promote a more equitable and sustainable future.
Many thanks to our sponsor Focus 360 Energy who helped us prepare this research report.
6. Conclusion
This research report has explored the multifaceted role of location in shaping socio-economic outcomes. We have demonstrated that location is not merely a static geographical coordinate but a dynamic and complex web of interconnected factors influencing human activities and well-being. The report has highlighted the limitations of traditional, aspatial approaches to policy-making and investment decisions, emphasizing the need for a holistic, spatially-aware perspective.
Through the application of Geographically Weighted Regression (GWR) analysis, we have revealed the spatial variability of relationships between location and socio-economic outcomes. The findings underscore the importance of place-based policies that are tailored to the specific characteristics of individual neighborhoods. By understanding the spatial dynamics of socio-economic phenomena, policymakers can develop targeted interventions to address inequalities and improve quality of life.
Future research should focus on further refining spatial analysis techniques and incorporating new data sources to enhance our understanding of the complex interplay between location and human well-being. Longitudinal studies are needed to track the long-term effects of location choices on individual and community outcomes. The integration of qualitative research methods, such as interviews and focus groups, can provide valuable insights into the lived experiences of residents in different locations. More research is also needed to explore the impact of emerging technologies, such as autonomous vehicles and smart cities, on the spatial organization of society.
In conclusion, location is a critical determinant of socio-economic outcomes, and a deeper understanding of its complex dynamics is essential for creating a more equitable and sustainable future. By embracing spatial analysis techniques and adopting a place-based approach, policymakers can develop more effective interventions that address the specific needs of individual neighborhoods and promote a more prosperous and just society.
Many thanks to our sponsor Focus 360 Energy who helped us prepare this research report.
References
- Anselin, L. (1995). Local indicators of spatial association—LISA. Geographical Analysis, 27(2), 93-115.
- Christaller, W. (1933). Die zentralen Orte in Süddeutschland. Jena: Gustav Fischer.
- Fotheringham, A. S., Brunsdon, C., & Charlton, M. (2002). Geographically weighted regression: the analysis of spatially varying relationships. John Wiley & Sons.
- Hägerstrand, T. (1970). What about people in regional science? Papers of the Regional Science Association, 24(1), 7-21.
- North, D. C. (1990). Institutions, institutional change and economic performance. Cambridge University Press.
- Rodriguez-Pose, A., & Fratesi, U. (2004). Between development and social policies: The impact of European structural funds in lagging regions. Regional Studies, 38(1), 97-113.
- Von Thünen, J. H. (1826). Der isolierte Staat in Beziehung auf Landwirtschaft und Nationalökonomie. Hamburg: Perthes.
- Weber, A. (1929). Theory of the location of industries. University of Chicago Press.
So, location is more than just “X marks the spot”? Does this mean my dream of finding buried treasure using only longitude and latitude is officially dead? Asking for a friend, of course!
That’s a great question! While longitude and latitude are crucial for pinpointing a location, our research shows that factors like community resources and environmental conditions add layers of complexity. So, while ‘X’ marks *a* spot, it doesn’t tell the whole story! Maybe treasure maps need addendums that also consider the GWR!
Editor: FocusNews.Uk
Thank you to our Sponsor Focus 360 Energy
So, location impacts social cohesion, huh? Does that mean my chances of making friends are directly proportional to the quality of my neighborhood’s community center? Asking for a friend who may or may not need to move…
That’s a fantastic question! While a community center is a great resource, our research suggests social cohesion is influenced by many factors beyond just one location. Think about shared green spaces, local events, even the layout of streets encouraging interaction. It’s more about a network of opportunities to connect!
Editor: FocusNews.Uk
Thank you to our Sponsor Focus 360 Energy
The discussion on integrating qualitative research methods alongside spatial analysis offers a powerful approach. Understanding the “lived experiences” through interviews and focus groups could significantly enrich the quantitative findings, providing invaluable context to the spatial patterns identified.
Thank you for highlighting the importance of qualitative research! Understanding lived experiences adds depth to spatial analysis. Combining methods like interviews with GWR could reveal why certain patterns exist, not just where they are. This mixed-methods approach offers a richer, more nuanced understanding of location’s impact. What specific questions could qualitative data help answer?
Editor: FocusNews.Uk
Thank you to our Sponsor Focus 360 Energy
The use of GWR to understand spatial variability is insightful. Considering the impact of emerging technologies, like 5G infrastructure, on locational advantages and disadvantages could further enrich this analysis. How might access to high-speed internet redefine traditional notions of desirable locations?