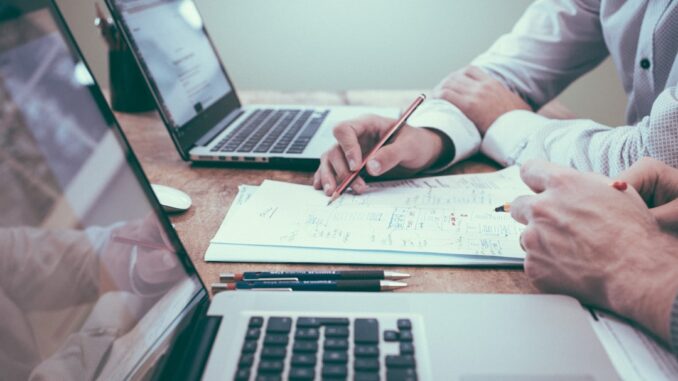
Abstract
Data siloing, the segregation of data within an organization or across interconnected systems, presents a significant impediment to effective decision-making, process optimization, and innovation. While frequently discussed within the context of business intelligence and customer relationship management, its pervasive impact extends far beyond these domains. This research report delves into the multifaceted nature of data siloing, examining its root causes, diverse manifestations across various sectors (including construction, healthcare, and finance), and the consequences for organizational performance and societal well-being. We explore a range of mitigation strategies, encompassing technological solutions (data integration platforms, APIs, federated databases), organizational practices (data governance frameworks, cross-functional collaboration), and cultural shifts (promoting data literacy and a data-driven mindset). Furthermore, the report critically examines the ethical implications of data siloing and data integration, addressing issues of privacy, bias, and algorithmic accountability. Our analysis reveals that overcoming data siloing requires a holistic approach that combines technological advancements with robust governance structures and a deep understanding of the ethical responsibilities associated with data management in an increasingly interconnected world.
Many thanks to our sponsor Focus 360 Energy who helped us prepare this research report.
1. Introduction
In today’s digital landscape, data is often hailed as the new oil, a valuable resource capable of fueling innovation, driving efficiency, and informing strategic decisions. However, the potential of data is frequently hampered by the pervasive problem of data siloing. Data siloing occurs when data is isolated within specific departments, applications, or systems, preventing seamless access, integration, and analysis across an organization or ecosystem. This fragmentation hinders a comprehensive understanding of complex phenomena, limits the ability to identify patterns and trends, and ultimately undermines the potential for data-driven insights.
While the consequences of data siloing are widely acknowledged, its root causes are often multifaceted and deeply embedded within organizational structures, technological infrastructure, and cultural norms. Legacy systems, departmental autonomy, a lack of data governance policies, and a reluctance to share information can all contribute to the creation and perpetuation of data silos. The impact of data siloing extends far beyond mere operational inefficiencies; it can impede innovation, hinder effective risk management, and even compromise ethical considerations related to data privacy and security.
This research report aims to provide a comprehensive exploration of data siloing, examining its manifestations across diverse domains, analyzing the challenges it poses, and proposing effective mitigation strategies. We will investigate the technological, organizational, and cultural factors that contribute to data siloing, and explore how these factors interact to shape the overall impact of data fragmentation. Furthermore, we will delve into the ethical implications of data siloing and data integration, considering the potential for unintended consequences and the importance of responsible data management practices.
Many thanks to our sponsor Focus 360 Energy who helped us prepare this research report.
2. The Roots and Manifestations of Data Siloing
Data siloing rarely arises spontaneously; it is typically the result of a confluence of factors, often intertwined and mutually reinforcing. Understanding these root causes is crucial for developing effective mitigation strategies.
2.1 Technological Factors:
- Legacy Systems: Many organizations rely on legacy systems that were designed in isolation and lack compatibility with modern data integration tools. These systems often use proprietary data formats and communication protocols, making it difficult to extract and integrate data with other platforms. The cost and complexity of replacing or upgrading these legacy systems can further exacerbate the problem.
- Lack of Interoperability: Different software applications and databases often use incompatible data formats and standards, preventing seamless data exchange. This lack of interoperability can arise from a lack of industry standards, competitive pressures between vendors, or simply the evolution of technology over time.
- Decentralized Data Storage: When data is stored in multiple locations and formats across an organization, it becomes difficult to maintain a consistent view of the data. Decentralized data storage can arise from departmental autonomy, a lack of centralized IT governance, or the use of cloud-based services without proper integration strategies.
2.2 Organizational Factors:
- Departmental Autonomy: Departments often operate independently, with their own budgets, priorities, and technology stacks. This can lead to the creation of data silos, as each department focuses on its own specific needs and neglects the broader organizational context. Furthermore, departments may be reluctant to share data with other departments, fearing a loss of control or competitive advantage.
- Lack of Data Governance: Without a clear data governance framework, there is no central authority responsible for defining data standards, ensuring data quality, and managing data access. This can lead to inconsistencies in data definitions, duplication of data, and a lack of trust in the data.
- Mergers and Acquisitions: Mergers and acquisitions often result in the integration of disparate systems and data sources, creating a complex and fragmented data landscape. Integrating these systems can be a challenging and time-consuming process, particularly if the organizations have different technology stacks and data management practices.
2.3 Cultural Factors:
- Lack of Data Literacy: Many employees lack the skills and knowledge to effectively use data in their decision-making processes. This can lead to a reluctance to embrace data-driven approaches and a preference for traditional methods of working.
- Fear of Change: Implementing data integration strategies can require significant changes to existing workflows and processes. Employees may resist these changes, fearing that they will lose their jobs or be forced to learn new skills.
- Lack of Trust: A lack of trust between departments or individuals can hinder data sharing and collaboration. This lack of trust may stem from past experiences, personality conflicts, or a perception that data sharing will disadvantage one party.
The manifestations of data siloing are diverse and vary depending on the specific context. However, some common examples include:
- Inconsistent Customer Data: Customer data may be stored in multiple systems, such as CRM, marketing automation, and customer support platforms. This can lead to inconsistent customer profiles and a fragmented view of the customer journey.
- Redundant Data Entry: Employees may be forced to enter the same data into multiple systems, leading to inefficiencies and errors.
- Delayed Decision-Making: Accessing and integrating data from multiple sources can be a time-consuming process, delaying decision-making and hindering responsiveness to changing market conditions.
- Missed Opportunities: Data silos can prevent organizations from identifying patterns and trends that could lead to new business opportunities or improved operational efficiency.
Many thanks to our sponsor Focus 360 Energy who helped us prepare this research report.
3. Data Siloing Across Diverse Domains: Case Studies
The impact of data siloing is not confined to any single industry or sector. Its consequences can be observed across a wide range of domains, each with its own unique challenges and opportunities. This section presents case studies illustrating the pervasive nature of data siloing and its impact on organizational performance and societal well-being.
3.1 Construction:
The construction industry is notoriously fragmented, with numerous stakeholders involved in each project, including architects, engineers, contractors, subcontractors, and suppliers. Each stakeholder typically uses its own software applications and data formats, leading to a complex web of data silos. This fragmentation can result in:
- Communication breakdowns: Lack of interoperability between different software systems can lead to miscommunication and delays, increasing the risk of errors and cost overruns.
- Inefficient workflows: Data may need to be manually transferred between different systems, leading to inefficiencies and wasted time.
- Difficulty in tracking project progress: A fragmented view of project data makes it difficult to track progress, identify potential problems, and make informed decisions.
3.2 Healthcare:
The healthcare industry is heavily regulated and relies on a vast amount of sensitive patient data. However, this data is often siloed across different healthcare providers, hospitals, and insurance companies. This fragmentation can result in:
- Difficulty in providing coordinated care: Lack of access to a complete patient history can make it difficult for healthcare providers to provide coordinated care, increasing the risk of medical errors and adverse events.
- Inefficient administrative processes: Claim processing and billing can be complicated by the need to access data from multiple sources.
- Limited opportunities for research and innovation: Data silos can prevent researchers from accessing the data they need to conduct studies and develop new treatments.
3.3 Finance:
The financial services industry relies on data for a wide range of purposes, including risk management, fraud detection, and customer relationship management. However, data is often siloed across different departments and business units, such as retail banking, investment banking, and insurance. This fragmentation can result in:
- Inability to detect fraudulent activity: Fraudulent transactions may go undetected if the data is not integrated across different systems.
- Difficulty in managing risk: Lack of a holistic view of risk can lead to inadequate risk management practices.
- Missed opportunities for cross-selling: Data silos can prevent financial institutions from identifying opportunities to cross-sell products and services to their customers.
These case studies highlight the diverse manifestations of data siloing and its potential consequences. Overcoming data siloing requires a tailored approach that takes into account the specific challenges and opportunities of each domain.
Many thanks to our sponsor Focus 360 Energy who helped us prepare this research report.
4. Mitigation Strategies: A Holistic Approach
Addressing data siloing requires a multi-faceted approach that encompasses technological solutions, organizational practices, and cultural shifts. A purely technological fix is unlikely to be successful without addressing the underlying organizational and cultural barriers. This section outlines a range of mitigation strategies, emphasizing the importance of a holistic perspective.
4.1 Technological Solutions:
- Data Integration Platforms: Data integration platforms provide a central hub for connecting disparate data sources and transforming data into a consistent format. These platforms typically offer a range of features, including data mapping, data transformation, and data quality management.
- APIs (Application Programming Interfaces): APIs enable different software applications to communicate with each other and exchange data. APIs can be used to integrate data from various sources in real-time, providing a more dynamic and up-to-date view of the data.
- ETL (Extract, Transform, Load) Processes: ETL processes are used to extract data from multiple sources, transform it into a consistent format, and load it into a data warehouse or data lake. ETL processes are typically used for batch processing of data, providing a historical view of the data.
- Federated Databases: Federated databases allow users to access data from multiple databases without having to physically move the data. This approach is useful when it is not feasible to consolidate data into a single database, for example, due to regulatory constraints or technical limitations.
- Data Virtualization: Data virtualization creates a virtual layer on top of existing data sources, allowing users to access and integrate data without having to move or transform the data. This approach is useful for accessing data from disparate sources in real-time.
4.2 Organizational Practices:
- Data Governance Framework: A data governance framework defines the policies, procedures, and responsibilities for managing data across an organization. The framework should address issues such as data quality, data security, data privacy, and data access.
- Cross-Functional Collaboration: Breaking down data silos requires collaboration between different departments and business units. This can be achieved through cross-functional teams, regular communication, and shared goals.
- Data Ownership and Stewardship: Assigning data ownership and stewardship responsibilities helps to ensure that data is accurate, complete, and consistent. Data owners are responsible for defining the data’s purpose and meaning, while data stewards are responsible for managing the data on a day-to-day basis.
- Data Catalog: A data catalog provides a central repository for metadata about data assets, including data definitions, data lineage, and data quality metrics. A data catalog helps users to discover and understand the data that is available to them.
4.3 Cultural Shifts:
- Promoting Data Literacy: Investing in data literacy training for employees helps to ensure that they have the skills and knowledge to effectively use data in their decision-making processes. This includes training on data analysis, data visualization, and data storytelling.
- Fostering a Data-Driven Mindset: Creating a culture that values data-driven decision-making is essential for breaking down data silos. This requires leadership buy-in, clear communication about the benefits of data-driven approaches, and recognition for employees who use data effectively.
- Encouraging Data Sharing: Breaking down data silos requires a willingness to share data across departments and business units. This can be achieved through incentives, recognition programs, and a culture of transparency.
By implementing these mitigation strategies, organizations can break down data silos and unlock the full potential of their data assets. The key is to adopt a holistic approach that addresses the technological, organizational, and cultural barriers to data integration.
Many thanks to our sponsor Focus 360 Energy who helped us prepare this research report.
5. Ethical Considerations: Navigating the Minefield
While data integration offers numerous benefits, it also raises significant ethical concerns. Integrating data from multiple sources can reveal sensitive information about individuals, potentially leading to privacy violations, discrimination, and other unintended consequences. It is crucial to address these ethical considerations proactively to ensure that data integration is used responsibly and ethically.
5.1 Privacy:
- Data Minimization: Collecting only the data that is necessary for a specific purpose helps to minimize the risk of privacy violations. Organizations should avoid collecting data that is not relevant to their stated purpose.
- Data Anonymization and Pseudonymization: Anonymizing or pseudonymizing data can help to protect the privacy of individuals. Anonymization removes all personally identifiable information from the data, while pseudonymization replaces personally identifiable information with pseudonyms.
- Data Security: Protecting data from unauthorized access is essential for maintaining privacy. Organizations should implement strong security measures, such as encryption, access controls, and regular security audits.
- Transparency: Being transparent about how data is collected, used, and shared helps to build trust with individuals. Organizations should provide clear and concise privacy policies that explain their data practices.
5.2 Bias:
- Data Bias: Data can reflect biases that are present in the real world. For example, data collected from a biased sample may lead to inaccurate or unfair conclusions. Organizations should be aware of the potential for data bias and take steps to mitigate it.
- Algorithmic Bias: Algorithms can also exhibit bias, particularly if they are trained on biased data. Organizations should carefully evaluate algorithms for bias and take steps to mitigate it.
- Fairness and Equity: Data integration should be used in a way that promotes fairness and equity. Organizations should avoid using data to discriminate against individuals or groups.
5.3 Algorithmic Accountability:
- Explainability: Algorithms should be explainable, meaning that it should be possible to understand how they work and why they make certain decisions. This is particularly important for algorithms that are used to make decisions that affect people’s lives.
- Auditability: Algorithms should be auditable, meaning that it should be possible to track their performance and identify potential problems. This requires clear documentation and logging of algorithm inputs, outputs, and decision-making processes.
- Responsibility: Organizations should be accountable for the decisions made by their algorithms. This requires establishing clear lines of responsibility and implementing mechanisms for redress when algorithms make mistakes.
Addressing these ethical considerations requires a commitment to responsible data management practices. Organizations should develop and implement ethical guidelines for data integration, train employees on ethical data practices, and establish mechanisms for monitoring and enforcing ethical compliance.
Many thanks to our sponsor Focus 360 Energy who helped us prepare this research report.
6. Conclusion
Data siloing represents a pervasive challenge across diverse domains, hindering organizational efficiency, impeding innovation, and raising significant ethical concerns. Overcoming this challenge requires a holistic approach that integrates technological solutions with robust data governance frameworks and a commitment to fostering a data-driven culture.
Technological advancements, such as data integration platforms, APIs, and federated databases, offer powerful tools for connecting disparate data sources and enabling seamless data access. However, these tools are only effective when implemented within a well-defined data governance framework that addresses issues such as data quality, data security, and data privacy.
Furthermore, breaking down data silos requires a cultural shift that promotes data literacy, encourages data sharing, and fosters a data-driven mindset. Organizations must invest in training employees on data analysis and visualization techniques, and create a culture that values data-driven decision-making.
The ethical considerations associated with data integration cannot be overlooked. Organizations must be proactive in addressing issues such as privacy, bias, and algorithmic accountability. This requires implementing ethical guidelines for data integration, training employees on ethical data practices, and establishing mechanisms for monitoring and enforcing ethical compliance.
In conclusion, overcoming data siloing is a complex and ongoing process that requires a commitment to continuous improvement. By adopting a holistic approach that addresses the technological, organizational, cultural, and ethical challenges, organizations can unlock the full potential of their data assets and create a more efficient, innovative, and ethical future.
Many thanks to our sponsor Focus 360 Energy who helped us prepare this research report.
References
- Beyer, M. A., & Laney, D. (2012). The importance of ‘big data’: A definition. Gartner. Retrieved from https://www.gartner.com/en/documents/2057415
- DAMA International. (2017). DAMA-DMBOK: Data Management Body of Knowledge (2nd ed.). Technics Publications.
- O’Reilly, T. (2007). What is Web 2.0: Design patterns and business models for the next generation of software. O’Reilly Media. Retrieved from https://www.oreilly.com/pub/a/web2/archive/what-is-web-20.html
- Shapiro, C., & Varian, H. R. (1998). Information rules: A strategic guide to the network economy. Harvard Business School Press.
- Manyika, J., Chui, M., Brown, B., Bughin, J., Dobbs, R., Roxburgh, C., & Byers, A. H. (2011). Big data: The next frontier for innovation, competition, and productivity. McKinsey Global Institute. Retrieved from https://www.mckinsey.com/~/media/mckinsey/business%20functions/mckinsey%20digital/our%20insights/big%20data%20the%20next%20frontier%20for%20innovation/mgi_big_data_full_report.pdf
- Floridi, L. (2014). The fourth revolution: How the infosphere is reshaping human reality. Oxford University Press.
- Zheng, Y., Capra, L., Wolfson, O., & Yang, H. (2014). Urban computing: concepts, methodologies, and applications. ACM Transactions on Intelligent Systems and Technology (TIST), 5(3), 1-55.
- O’Neil, C. (2016). Weapons of math destruction: How big data increases inequality and threatens democracy. Crown.
- Metcalf, J., Crawford, K., & Calo, R. (2016). The sociotechnical conditions of machine learning success and failure. Journal of Information Policy, 6, 369-398.
- Data & Society Research Institute. (n.d.). Algorithmic Accountability. Retrieved from https://datasociety.net/topic/algorithmic-accountability/
- European Union Agency for Fundamental Rights (FRA). (2019). Algorithms and Fundamental Rights. Publications Office of the European Union.
- World Economic Forum. (2019). Our Shared Digital Future: Building a Trustworthy, Inclusive and Sustainable Digital Society. World Economic Forum.
The ethical considerations around algorithmic accountability are particularly important. As data integration increases, how can organizations best ensure explainability and auditability in their algorithms to prevent unintended consequences and maintain public trust?