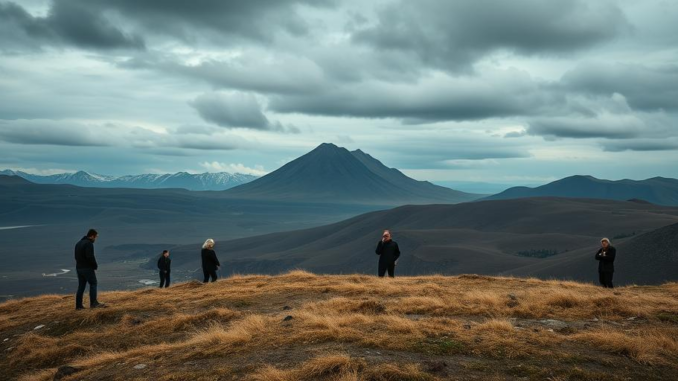
Abstract
This research report provides a comprehensive exploration of the concept of evidence across a range of disciplines, moving beyond its specific application within BREEAM assessments. It delves into the philosophical underpinnings of evidence, its role in legal systems, scientific inquiry, historical analysis, and increasingly, within the context of artificial intelligence and machine learning. By examining the epistemological foundations and practical applications of evidence in these diverse fields, the report aims to provide a nuanced understanding of its inherent complexities, challenges, and evolving interpretations. The analysis addresses questions of validity, reliability, interpretation, and bias in evidence evaluation, ultimately contributing to a more robust framework for understanding and utilizing evidence in various domains, including, by extension, sustainability assessments like BREEAM.
Many thanks to our sponsor Focus 360 Energy who helped us prepare this research report.
1. Introduction: Defining and Contextualizing Evidence
The concept of evidence is fundamental to human understanding and decision-making. While frequently associated with legal proceedings and scientific investigation, evidence underpins a far broader range of activities, including historical analysis, medical diagnosis, and increasingly, the operation of artificial intelligence systems. Defining evidence in a universally applicable manner, however, proves to be a complex undertaking. The Oxford English Dictionary defines evidence as “the available body of facts or information indicating whether a belief or proposition is true or valid.” This definition, while useful, glosses over the nuanced interpretations and debates surrounding what constitutes a “fact,” how to assess its “validity,” and the processes involved in “indicating” truth. Furthermore, the context within which evidence is considered significantly influences its interpretation and perceived value.
In legal contexts, evidence often refers to testimony, documents, or physical objects presented in court to prove or disprove a fact in dispute. The rules of evidence, which vary across jurisdictions, govern the admissibility and weight assigned to different types of evidence. Scientific evidence, on the other hand, relies heavily on empirical data obtained through experimentation and observation. The validity of scientific evidence is typically assessed through rigorous statistical analysis and peer review. Historical evidence comprises primary sources, such as letters, diaries, and official documents, as well as secondary sources that interpret and analyze these materials. Historians critically evaluate the authenticity and reliability of historical evidence, considering the potential biases and perspectives of the individuals or institutions that produced it.
The emergence of artificial intelligence (AI) and machine learning (ML) has introduced new dimensions to the concept of evidence. AI systems often rely on vast datasets to identify patterns and make predictions. The data used to train these systems can be considered a form of evidence, but its interpretation and impact are subject to ongoing debate. Issues of bias, transparency, and accountability are particularly relevant in the context of AI-driven decision-making, as the evidence used by these systems may not always be readily accessible or understandable.
This report argues that a comprehensive understanding of evidence requires a multi-disciplinary approach, drawing on insights from philosophy, law, science, history, and artificial intelligence. By examining the epistemological foundations and practical applications of evidence in these diverse fields, we can develop a more nuanced appreciation of its inherent complexities and challenges.
Many thanks to our sponsor Focus 360 Energy who helped us prepare this research report.
2. The Epistemology of Evidence: Justification and Belief
Epistemology, the branch of philosophy concerned with the nature of knowledge, provides a crucial framework for understanding the role of evidence in justifying beliefs. Traditional epistemology often focuses on the relationship between belief, truth, and justification. To be considered knowledge, a belief must be both true and justified. Evidence plays a critical role in providing this justification.
Different epistemological theories offer varying perspectives on the nature of justification and the role of evidence. Foundationalism, for example, posits that all justified beliefs ultimately rest on a foundation of basic, self-evident beliefs that do not require further justification. These basic beliefs often derive from sensory experience or logical intuition. In this framework, evidence serves to build a structure of justified beliefs upon this foundational base.
Coherentism, in contrast, emphasizes the coherence of a system of beliefs as the primary source of justification. According to coherentism, a belief is justified if it fits coherently with other beliefs within the system. Evidence, in this context, contributes to the overall coherence of the belief system. A piece of evidence that conflicts with existing beliefs may be rejected or reinterpreted to maintain coherence. This perspective highlights the importance of contextual factors in the interpretation of evidence.
Reliabilism focuses on the reliability of the processes that generate beliefs. According to reliabilism, a belief is justified if it is produced by a reliable belief-forming process. Evidence, in this framework, is valuable insofar as it is generated by a reliable process. For example, scientific evidence obtained through well-designed experiments and rigorous statistical analysis is considered more reliable than anecdotal evidence or personal intuition.
These epistemological theories provide different perspectives on the nature of justification and the role of evidence. However, they all recognize the importance of evidence in supporting and validating beliefs. The specific type of evidence that is considered relevant and the criteria used to evaluate its validity may vary depending on the epistemological framework and the context in which it is being considered.
Many thanks to our sponsor Focus 360 Energy who helped us prepare this research report.
3. Evidence in Legal Systems: Admissibility and Weight
Legal systems around the world rely heavily on evidence to determine guilt or innocence in criminal cases and to resolve disputes in civil cases. The rules of evidence, which vary across jurisdictions, govern the admissibility and weight assigned to different types of evidence. These rules are designed to ensure fairness, reliability, and due process.
Admissibility refers to the criteria that evidence must meet in order to be presented in court. Common rules of admissibility include relevance, authenticity, and reliability. Relevant evidence is evidence that tends to prove or disprove a fact in dispute. Authentic evidence is evidence that is what it purports to be. Reliable evidence is evidence that is trustworthy and accurate.
There are several types of evidence commonly used in legal proceedings. Direct evidence is evidence that directly proves a fact in dispute without requiring any inferences. Eyewitness testimony is an example of direct evidence. Circumstantial evidence, on the other hand, is evidence that indirectly proves a fact in dispute by requiring the fact-finder to draw inferences. Fingerprints, DNA evidence, and motive are examples of circumstantial evidence.
The weight of evidence refers to the degree of persuasiveness that a particular piece of evidence has. The weight of evidence is determined by the fact-finder (judge or jury) based on their assessment of its credibility, relevance, and reliability. Factors that can affect the weight of evidence include the source of the evidence, the method by which it was obtained, and the potential biases of the individuals involved.
Challenges in evaluating evidence in legal systems include issues of witness credibility, the admissibility of scientific evidence, and the potential for bias. Witness testimony can be unreliable due to memory distortions, suggestibility, and intentional deception. Scientific evidence, such as DNA evidence and forensic analysis, must be carefully scrutinized to ensure that it is valid and reliable. Bias can arise from a variety of sources, including personal prejudices, institutional biases, and selective presentation of evidence.
Many thanks to our sponsor Focus 360 Energy who helped us prepare this research report.
4. Evidence in Scientific Inquiry: Observation, Experimentation, and Peer Review
Scientific inquiry relies on empirical evidence to test hypotheses and develop theories. The scientific method, which emphasizes observation, experimentation, and data analysis, is designed to generate reliable and valid evidence. Scientific evidence is typically quantitative, based on measurements and statistical analysis, but can also include qualitative data derived from observations and interviews.
Observation involves systematically gathering information about the natural world through the senses or through the use of scientific instruments. Observations can be qualitative (e.g., describing the color of a flower) or quantitative (e.g., measuring the temperature of a solution). Experimentation involves manipulating variables to determine cause-and-effect relationships. A well-designed experiment includes a control group, which does not receive the treatment, and an experimental group, which does receive the treatment. By comparing the outcomes of the two groups, researchers can determine whether the treatment has a significant effect.
Statistical analysis is used to analyze data and determine the probability that the results are due to chance. Statistical significance is typically defined as a p-value of less than 0.05, meaning that there is a less than 5% chance that the results are due to chance. Peer review is a process by which scientific research is evaluated by other experts in the field before it is published in a scientific journal. Peer review helps to ensure the quality and validity of scientific research.
Challenges in evaluating scientific evidence include issues of reproducibility, bias, and the interpretation of statistical data. Reproducibility refers to the ability of other researchers to replicate the results of a study. A lack of reproducibility can indicate problems with the methodology, data analysis, or interpretation of the results. Bias can arise from a variety of sources, including researcher bias, funding bias, and publication bias. The interpretation of statistical data can be challenging, especially when dealing with complex datasets or small sample sizes. The misuse or misinterpretation of statistics can lead to erroneous conclusions.
Many thanks to our sponsor Focus 360 Energy who helped us prepare this research report.
5. Evidence in Historical Analysis: Primary and Secondary Sources, Interpretation and Bias
Historical analysis relies on a variety of sources to reconstruct and interpret the past. Primary sources are firsthand accounts or artifacts from the period being studied. Examples of primary sources include letters, diaries, official documents, photographs, and archaeological artifacts. Secondary sources are interpretations and analyses of primary sources written by historians or other scholars. Examples of secondary sources include books, articles, and documentaries.
Historians critically evaluate the authenticity and reliability of historical evidence. They consider the source of the evidence, the context in which it was created, and the potential biases of the individuals or institutions that produced it. Historical evidence is often incomplete or fragmented, requiring historians to piece together a coherent narrative from disparate sources.
Interpretation plays a crucial role in historical analysis. Historians must interpret the meaning and significance of historical evidence in light of its historical context. Different historians may offer different interpretations of the same evidence, leading to debates and controversies. Bias is a significant challenge in historical analysis. Historians may be influenced by their own personal beliefs, cultural values, or political agendas. It is important to recognize and account for potential biases when evaluating historical evidence.
Examples of bias in historical evidence include the selective preservation of documents, the distortion of events in official accounts, and the perpetuation of stereotypes and prejudices in popular narratives. Historians strive to overcome bias by consulting a wide range of sources, critically evaluating the perspectives of different authors, and acknowledging the limitations of their own interpretations.
Many thanks to our sponsor Focus 360 Energy who helped us prepare this research report.
6. Evidence in Artificial Intelligence and Machine Learning: Data, Algorithms, and Transparency
The increasing reliance on artificial intelligence (AI) and machine learning (ML) systems raises important questions about the nature of evidence in these contexts. AI systems often rely on vast datasets to identify patterns and make predictions. The data used to train these systems can be considered a form of evidence, but its interpretation and impact are subject to ongoing debate. Algorithms, which are the sets of rules that guide AI systems, also play a crucial role in determining how evidence is processed and used.
Data quality is a critical factor in the performance and reliability of AI systems. Biased or incomplete data can lead to inaccurate or unfair outcomes. For example, if an AI system is trained on data that disproportionately represents one demographic group, it may produce biased results when applied to other groups. Ensuring data quality requires careful attention to data collection, cleaning, and validation procedures.
The transparency of AI algorithms is also a significant concern. Many AI systems, particularly those based on deep learning, are “black boxes,” meaning that it is difficult to understand how they arrive at their decisions. This lack of transparency can make it challenging to identify and correct biases or errors in the system. Explainable AI (XAI) is a growing field that aims to develop AI systems that are more transparent and understandable.
Ethical considerations are paramount in the development and deployment of AI systems. Issues of privacy, fairness, accountability, and transparency must be addressed to ensure that AI is used responsibly and ethically. The use of AI in sensitive areas, such as criminal justice, healthcare, and education, requires careful scrutiny and oversight.
The challenges in evaluating evidence in AI systems include the sheer volume of data involved, the complexity of the algorithms, and the potential for unintended consequences. Developing robust methods for auditing and validating AI systems is essential to ensure their safety and reliability.
Many thanks to our sponsor Focus 360 Energy who helped us prepare this research report.
7. Conclusion: A Unified Framework for Evaluating Evidence
This report has explored the concept of evidence across a range of disciplines, highlighting its complexities and challenges. While the specific types of evidence and the criteria used to evaluate it may vary depending on the context, several common themes emerge.
First, the interpretation of evidence is always context-dependent. The meaning and significance of a piece of evidence can only be understood in relation to its historical, social, and cultural context. Second, bias is a pervasive challenge in the evaluation of evidence. Bias can arise from a variety of sources, including personal prejudices, institutional biases, and selective presentation of evidence. It is important to recognize and account for potential biases when evaluating evidence.
Third, transparency and accountability are essential for ensuring the integrity of evidence-based decision-making. The methods used to collect, analyze, and interpret evidence should be transparent and open to scrutiny. Individuals and institutions should be held accountable for the accuracy and reliability of the evidence they present.
Finally, a multi-disciplinary approach is crucial for developing a comprehensive understanding of evidence. By drawing on insights from philosophy, law, science, history, and artificial intelligence, we can develop a more nuanced appreciation of the challenges and opportunities associated with evidence-based decision-making.
In the context of BREEAM assessments, this broader understanding of evidence informs a more critical and robust approach to evidence collection and evaluation. Understanding the potential for bias in documentation, the importance of contextual factors in interpreting performance data, and the limitations of relying solely on quantifiable metrics are all essential for ensuring the validity and reliability of BREEAM certifications. Furthermore, lessons learned from AI’s struggles with data bias can inform better practices in ensuring BREEAM assessments are equitable and representative of real-world performance.
Many thanks to our sponsor Focus 360 Energy who helped us prepare this research report.
References
- Ayer, A. J. (1936). Language, Truth and Logic. Victor Gollancz Ltd.
- Goldman, A. I. (1979). What is justified belief? In G. Pappas (Ed.), Justification and knowledge (pp. 1-23). D. Reidel Publishing Company.
- Haack, S. (2009). Evidence Matters: Science, Proof, and Truth in the Law. Cambridge University Press.
- Hempel, C. G. (1965). Aspects of Scientific Explanation. Free Press.
- Kuhn, T. S. (1962). The Structure of Scientific Revolutions. University of Chicago Press.
- Popper, K. R. (1959). The Logic of Scientific Discovery. Hutchinson & Co.
- Toulmin, S. E. (1958). The Uses of Argument. Cambridge University Press.
- United States Federal Rules of Evidence.
- O’Neil, C. (2016). Weapons of Math Destruction: How Big Data Increases Inequality and Threatens Democracy. Crown.
- Rudin, C. (2019). Stop explaining black box machine learning models for high stakes decisions and use interpretable models instead. Nature Machine Intelligence, 1(5), 206-215.
AI’s reliance on data as “evidence” is fascinating. But if AI was a detective, would it trust eyewitness testimony, or only cold, hard numbers? Could we teach it to spot a red herring? Perhaps a new branch of “AI forensics” is in order!
That’s a great point! “AI Forensics” is a compelling idea. The challenge of teaching AI to evaluate different types of evidence, especially subjective things like eyewitness accounts or identifying red herrings, is definitely a hurdle. It highlights the need for AI to develop a more nuanced understanding of context and potential biases in data.
Editor: FocusNews.Uk
Thank you to our Sponsor Focus 360 Energy
The report’s point about context-dependent interpretation of evidence is critical, especially when considering historical analysis. How can we develop methodologies to account for the potential loss of contextual understanding over time, ensuring that interpretations remain as accurate as possible?
That’s a vital question! Developing methodologies is key. Perhaps a blend of interdisciplinary approaches, including detailed source criticism frameworks and collaborative efforts between historians and experts in related fields like anthropology and linguistics, could help mitigate that loss of contextual understanding over time. What are your thoughts?
Editor: FocusNews.Uk
Thank you to our Sponsor Focus 360 Energy
The report mentions context-dependent interpretation across disciplines. In AI and machine learning, how can we ensure the “context” used by algorithms aligns with human understanding and values, preventing unintended or ethically questionable outcomes?
That’s a crucial question! The report highlighted that point. We can explore more nuanced training data sets, incorporating elements of human value judgements. This could involve collaborative feedback loops with diverse groups to refine the AI’s understanding of context and to help it identify ethically questionable outcomes.
Editor: FocusNews.Uk
Thank you to our Sponsor Focus 360 Energy